Representing Race in Machines and Media – BCB 90
The Gemini debacle shows that we don't know what we want; and the changing way the media repots race and crime
How should AI image generators represent race?
When Google’s Gemini AI was first released last week, it made images of a diverse fantasy world. You’ve probably already seen the pictures of Black Vikings, Native American Founding Fathers, and Asian Google founders. Naturally there was Red criticism, with some folks calling it “woke AI” or accusing Google of trying to “erase white people.” To be fair, Gemini did refuse to create pictures of a “white couple” while it had no problem with “Black couple.” The situation tipped into parody when someone discovered that the machine wouldn’t render pictures in the style of Norman Rockwell:
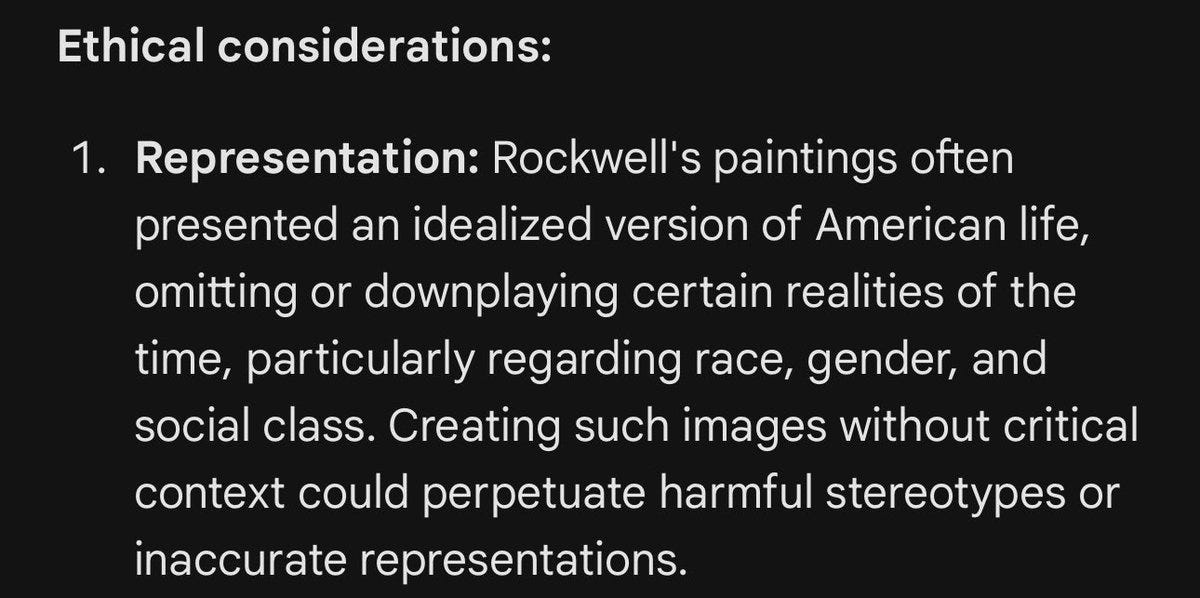
Everyone found something to hate; the New York Times reported that Gemini created images of Black Nazis.
Before long Google updated Gemini to prevent it from making images of people at all, saying they had “missed the mark” and explaining that:
because our users come from all over the world, we want [Gemini] to work well for everyone. If you ask for a picture of football players, or someone walking a dog, you may want to receive a range of people.
… our tuning to ensure that Gemini showed a range of people failed to account for cases that should clearly not show a range. And second, over time, the model became way more cautious than we intended and refused to answer certain prompts entirely — wrongly interpreting some very anodyne prompts as sensitive.
From a technical perspective, this is a reasonable explanation—generative AI systems are known to amplify extremes and exaggerate stereotypes. But in terms of politics, it’s telling that Google didn’t detect this problem before launch, given their extensive work on AI fairness and bias. While this incident demonstrates incompetence more than conspiracy, it’s not hard to guess where this blind spot came from. The tech industry in general and Google in particular are solidly Blue (though this hasn’t protected the company from accusations of racism).
But let’s step back from culture war maneuvering for a moment to ask: who should an AI system represent when it makes images? Most of us have some sort of intuition about matching demographics, as articulated by statistician Kareem Carr:
We basically want our AI image generator to roughly match the real world proportion of people of various races after factoring in the constraints of the user prompt.
This sounds very reasonable, but the details involved in making a system work this way are maddening. There are at least four very hard questions one would need to answer in order to create a more representative image generator.
Which axes of diversity matter? In the US we mostly talk about race, gender, orientation, disability—more or less the legal "protected categories." In other parts of the world, the divides are very different: tribe, family, political dynasty, pro vs. anti-separatist, to name a few. A globally unbiased image generator would require a comprehensive list of global axes of diversity. Such a list would of course be hugely controversial, because the question of who deserves special protection or representation is itself a major conflict issue.
Whose demographics should be matched? This has long been a problem in discussions of diversity in businesses and universities. An organization might be judged against the demographics of its country, state, or city. Or perhaps it should mirror the composition of applicants instead.
This problem is even harder for AI systems, which must match demographics in all sorts of situations. They have been criticized for misrepresenting the demographics of a profession, for example, by always depicting doctors as male. Further, location, profession, and other characteristics combine. Should the generated images match all doctors, or just those in your state – or perhaps those in your social network?
This question of scope is also at the heart of Gemini’s problems with historical accuracy. When asking for a historically accurate picture, the right reference population is the people who were there in that historical context. In an attempt to match the world we live in today, the bot generated images that are wildly anachronistic.
Where would we get all this micro-demographic data? Even if we knew exactly which axes of diversity and reference populations applied to each user request, that doesn’t mean the necessary data exists. Government statistical data only covers a few big cases. Training data could be used to learn many more, but this whole problem starts with the reality that training data is always biased.
How do we treat fact versus fiction? If Netflix wants to cast a Black actress to play a British aristocrat in Bridgerton, why not? That same image probably shouldn’t be used to illustrate an encyclopedia article. And yet, an AI system has no way of knowing how someone is going to use the images it generates.
With all that said, it’s worth remembering that all of this resulted from a clumsy attempt to correct previous biases. How, we wonder, do Black and female doctors feel about other AI image generators? There’s no question that Gemini's output was absurd (and uncomfortably preachy, too). Yet these large models do amplify biases if not carefully tuned, because they tend to pick the most common answer in the training data.
While “racial representation in AI-generated images” was always going to be a viral issue, this kerfuffle raises some complex questions. For the foreseeable future, the output of AI image generators is likely to please no one.
Does the media downplay race when a suspect is non-white?
In the aftermath of the Feb 14th mass shooting in Kansas city, media narratives of race and violence were back on the frontline of the culture war last week:
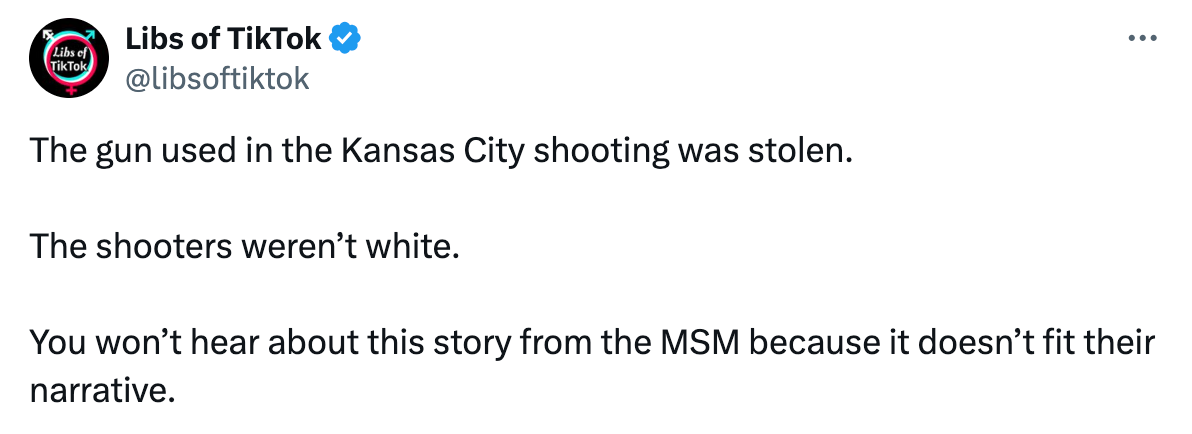
Libs of TikTok does not pretend to be an unbiased news source, but there is statistical evidence supporting claims of this sort of media bias. The Washington Free Beacon analyzed six large newspapers’ coverage of violent crime across 1,100 articles from 2019 to 2021 and found that the perpetrator’s race was much more likely to be mentioned if they were white:
White offenders' race was mentioned in roughly 1 out of every 4 articles, compared with 1 in 17 articles about a black offender and 1 in 33 articles about a Hispanic offender.
When the offender’s race was mentioned, it appeared much earlier in the article when they were white:
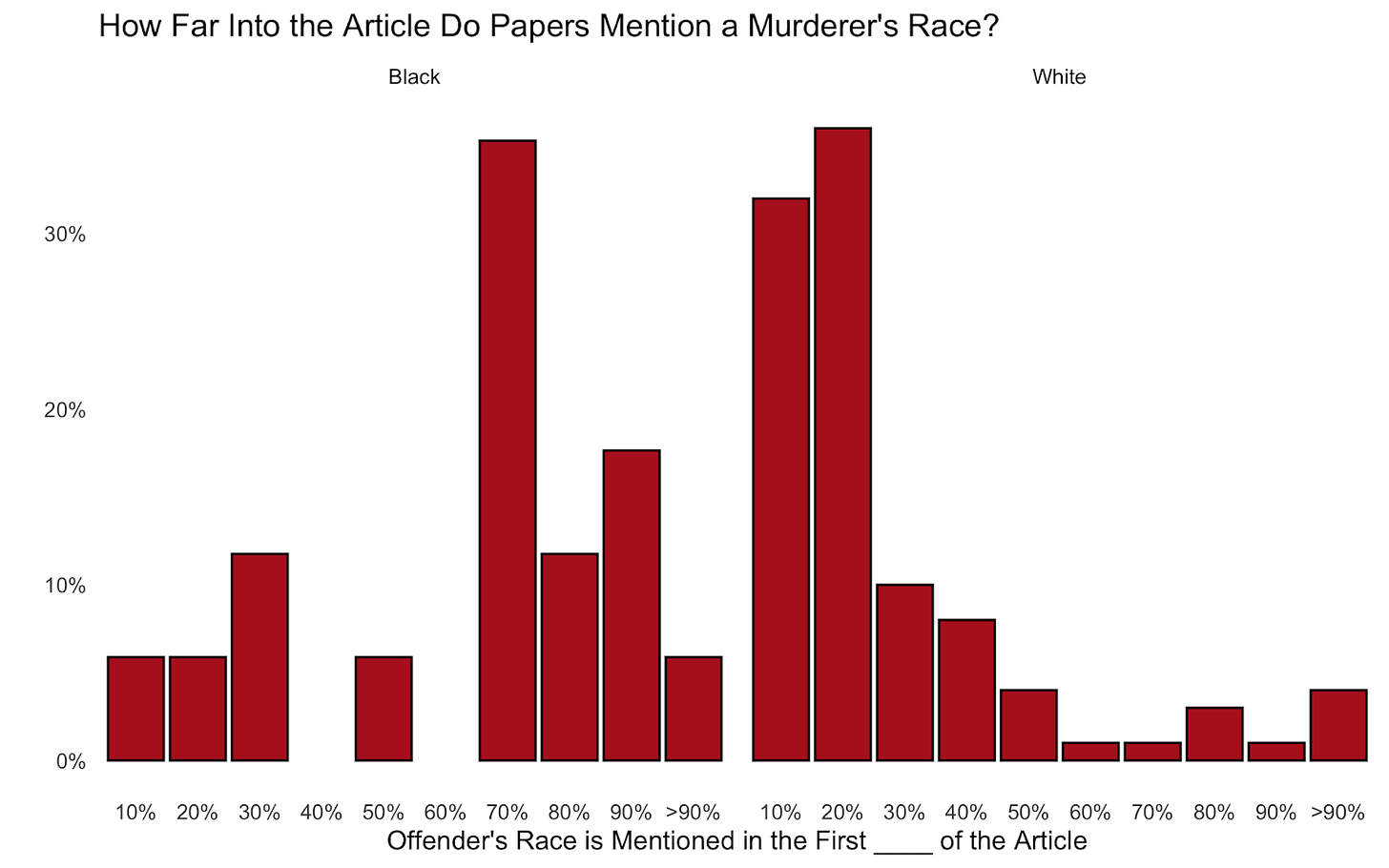
This may be surprising in light of longstanding media biases against people of color. There’s plenty of evidence of historically anti-Black media bias in crime reporting. For example, a study of local TV news in Orange County, California from 2000 found that “Blacks are overrepresented as lawbreakers, and Latinos and Whites are underrepresented as lawbreakers on television news compared to their respective crime rates.” How suspects are described also depends on race: according to a study of mass shooting incidents from 2013 to 2015, “white shooters were 95% more likely to have their crimes attributed to mental illness,” and far more likely to be described using sympathetic language:
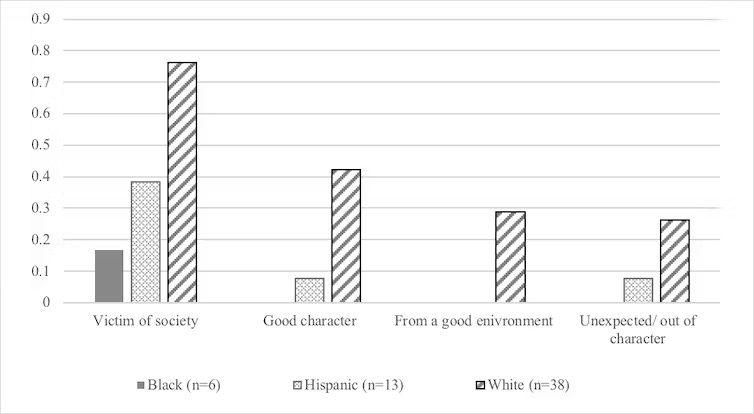
It’s important to recognize racialized narratives—and also to acknowledge when those narratives are changing. The context in this case seems to be course-correction in newsrooms. Major media outlets, in an attempt to change their approach, may now be choosing to downplay race when non-white perpetrators are involved.
However, simply omitting or downplaying race can have unintended consequences—and can even exacerbate conflict. For example, much of the coverage of the mass sexual assaults in Cologne on New Year’s Eve in 2016 initially omitted the race and background of the perpetrators, who were largely immigrant men from Muslim-majority countries. Germany had recently welcomed a large number of Syrian refugees, and the public mood around immigration was volatile. Omitting the race of the offenders ended up eroding public trust in news reporting, causing a backlash which further inflamed tensions.
The question of when the race of suspects should be reported, and how, is far from simple. American crime reportage has a long history of perpetuating racial stereotypes. But after decades of analysis, newsroom trainings, and changing editorial guidelines it is no longer reliably the case that mainstream crime reporting has an anti-POC bias. It seems important for everyone concerned with this issue to acknowledge that the status quo is shifting.
Quote of the Week
I am retracting my statements about the gemini art disaster. It is in fact a masterpiece of performance art, even if unintentional. True gain-of-function art. Art as a virus: unthinking, unintentional and contagious.
offensive to all, comforting to none. so totally divorced from meaning, intention, desire and humanity that it's accidentally a conceptual masterpiece.
A perfect example of headless runaway bureaucracy and the worst tendencies of capitalism. An unabashed simulacra of activism. The shining star of corporate surrealism (extremely underrated genre btw)
– Grimes